Embracing the Artificial Intelligence Green Revolution: Unleashing Innovation in Plant Sciences
Why does AI matter in this verdant domain? Picture a world where data dances with genomes, phenotypes, and environmental variables in an intricate waltz of insight. AI orchestrates this symphony, revealing patterns and predicting outcomes at a speed that would make Mendel’s peas blush. From spotting disease outbreaks to sculpting resilient crops, AI stands as the silent gardener, tending to our botanical bounty with digital finesse. In the lush fields of plant sciences, a digital dawn is breaking. Our recent survey journeyed into the minds of researchers, unveiling a tapestry of perceptions on the rising star: artificial intelligence (AI). Amidst a chorus of moderate awareness, AI’s prowess in disease detection, phenotyping, and breeding echoed as promising melodies. Yet, like tendrils reaching for the sun, doubts lingered about trust, ethics, literacy, and the specter of obsolescence. Tools like ChatGPT and GitHub Copilot wove through this landscape, painting a portrait of AI’s multifaceted role in cultivating greener pastures.
There is a need to raise awareness among people about the significant impact that AI can have on advances in plant science. Our survey encompasses diverse participants, including undergraduates, postgraduates, PhD students, postdocs, data scientists, and individuals from many other backgrounds worldwide. Merely 8% of respondents claimed to possess a high level of familiarity with the subject matter, while a significant majority of 62% reported having a moderate level of familiarity and said that they have a basic understanding of what it does. Currently, there is an assumption that AI has the capacity to influence advancements in fields like genomics and phenotyping. However, most people are unsure about the extent to which it will be beneficial for practical use in the field of plant science. Although many people are not conversant about integrating AI with plant science, it promises to revolutionize all areas of scientific research. Many researchers agreed that AI has the potential to substantially improve the precision of plant breeding, early detection of disease, yield prediction, climate modeling, and plant phenotyping, sparking hope for a more efficient and sustainable future in plant science.
This article addresses the impact of AI on advancements in plant research, the ethical implications of its use, concerns for ethical practices, challenges faced by plant scientists when incorporating AI into their workflows, and the prospects of AI in plant science. To optimize the advantages of AI for plant scientists, we put forward the following succession plan:
- Building Trust in AI Results
Challenge: Many respondents indicated they only sometimes trust AI results.
Solution:
Transparency and Explainability (XAI): Implementing explainable AI (XAI) methods is crucial. XAI makes AI models more interpretable, allowing researchers to understand the decision-making process. This transparency builds trust and facilitates the adoption of AI in plant sciences. XAI techniques include visualizing feature importance, generating model-agnostic explanations, and employing surrogate models to explain complex AI models.
Validation through Field Trials: Extensive validation through field trials can enhance trust. Montesinos-López et al, (2021) demonstrated that cross-validation techniques, such as k-fold cross-validation, alongside real-world testing, provide robust evidence of an AI model’s reliability. For example, predicting crop yield through deep learning models was validated against multiple seasons of field data, ensuring the model’s predictions were consistent and accurate.
- Ethical Guidelines and Bias Mitigation
Challenge: Ethical concerns regarding AI guidelines and algorithmic biases.
Solution:
Developing Ethical Frameworks: Establishing comprehensive ethical guidelines is essential. Floridi et al, (2018) outline principles such as transparency, accountability, and fairness, which are vital for ethical AI use. Transparency involves making AI processes and decisions understandable to users. Accountability ensures that the use of AI can be audited and that users are responsible for AI outcomes. Fairness means that AI systems should not perpetuate or amplify biases, and efforts should be made to ensure equitable treatment for all groups.
Bias Mitigation with Fairness-Aware Machine Learning: Ensuring datasets are diverse and representative can mitigate biases in AI algorithms. Fairness-aware machine learning techniques involve algorithms designed to identify and correct biases during model training. These methods can include reweighting training data, modifying learning objectives to include fairness constraints, and post-processing predictions to adjust for any detected biases, as mentioned in (Mehrabi et al, 2021).
Ethics Training: Incorporating ethics training in AI education can foster a culture of responsibility among researchers. Ethics training can include case studies, practical exercises, and discussions on the societal impacts of AI. Courses such as “AI Ethics: Implications and Responsibility” on Coursera and “Responsible AI” by IBM on edX provide foundational knowledge on ethical AI use. Ethic training has been further elaborated by (Mittelstadt et al, 2016) and (Floridi et al, 2018).
- Enhancing AI Literacy and Addressing Data Privacy
Challenge: Concerns about AI literacy and data privacy.
Solution:
Educational Resources: Bridging the AI literacy gap through free educational resources is imperative. Platforms like Coursera, edX, and YouTube offer comprehensive AI and machine learning courses. Notable AI experts like Andrew Ng provide free lessons on platforms like YouTube, making AI accessible to a broader audience. Other valuable resources include “Deep Learning Specialization” by Andrew Ng on Coursera and “AI For Everyone” by Andrew Ng.
Data Privacy Measures: Adhering to stringent data governance policies can address privacy concerns. Implementing data anonymization, secure data storage, and clear consent protocols can protect data privacy while enabling AI research. The European Union’s General Data Protection Regulation (GDPR) by Voigt and Von dem Bussche (2017) provides a robust framework for data privacy that can be adapted for AI research in plant sciences.
Community Outreach: Engaging with agricultural communities and stakeholders through workshops and training sessions can enhance AI literacy. Community-driven initiatives can demystify AI and promote its practical applications in plant sciences. For instance, the AI for Good Global Summit of 2021 by the International Telecommunication Union (ITU) brings together AI experts and community stakeholders to explore AI applications and challenges.
- Ensuring AI’s Relevance Amidst Evolving Technologies
Challenge: AI might either revolutionize or become obsolete in plant science due to new technologies.
Solution:
Adaptability and Integration: AI’s adaptability is its strength. Integrating emerging technologies such as quantum computing, advanced robotics, and blockchain can keep AI relevant and enhance its capabilities. For instance, quantum computing can solve complex optimization problems in plant breeding, while advanced robotics can automate phenotyping processes (Harfouche et al, 2023). For example, Google’s Sycamore processor has demonstrated quantum supremacy, showing potential for solving complex problems faster than classical computers. The University of Illinois has developed a robot named “TerraSentia” that uses AI to autonomously phenotype plants. Projects like “AgriChain” use blockchain to enhance transparency and traceability in agricultural supply chains.
Interdisciplinary Approaches: Promoting multidisciplinary research is crucial. A review by Jordan and Mitchell (2015) emphasizes that combining AI with other scientific fields can lead to innovative solutions and sustained relevance. For example, combining AI with molecular biology has led to breakthroughs in gene editing and crop improvement.
- Promoting Applications in Plant Disease Detection and Phenotyping
Challenge: While AI’s potential in plant disease detection and phenotyping is acknowledged, practical application needs scaling.
Solution:
Collaborative Projects: Enhancing collaborative efforts between academia, industry, and agricultural communities can expedite AI deployment. Singh et al, (2018) highlight successful projects like the use of machine learning for high-throughput phenotyping and stress detection in plants, which can be scaled for broader applications. Examples include the Breeding Insight project at Cornell University, which integrates AI and genomics for plant breeding.
Open-Access Platforms and Datasets: Developing open-access platforms and community-driven projects can facilitate widespread adoption. Platforms like PlantCV, a free, open-source image analysis software for plant phenotyping, and datasets from initiatives such as the European Plant Phenotyping Network (EPPN) and Terraref provide valuable resources for researchers (Schuhl et al, 2022).
Field Implementations: Conducting large-scale field implementations and pilot projects can demonstrate AI’s practical benefits. Sharing success stories and case studies can inspire broader acceptance and use of AI in plant sciences.
Conclusion: AI is poised to revolutionize plant sciences; it’s a powerful force that enables us to reconsider how we integrate information, analyze data, and utilize the resulting knowledge to enhance decision-making. The potential of this technology in plant science is enormous, and it could completely transform our understanding and interaction with plants, yet its journey is filled with challenges. We can harness AI’s full potential by addressing current challenges, establishing ethical guidelines, enhancing AI literacy, ensuring relevance, and promoting practical applications. Transparency, interdisciplinary research, and community engagement are crucial for overcoming these challenges. The future of plant sciences hinges on our ability to integrate AI responsibly, fostering a new era of innovation and sustainability.
Acknowledgments: We sincerely thank all the participants in the Survey for providing their suggestions for a greater understanding of how AI tools can revolutionize the field of plant science.
Further Reading and Learning Resources
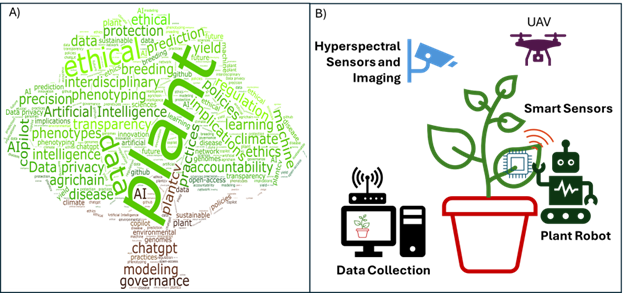
Figure 1: A) Wordcloud showing AI tools can revolutionize the field of plant science. B) Illustration showing AI-trained robotics devices can boost Innovation in farming.
References
Floridi, L., Cowls, J., Beltrametti, M., Chatila, R., Chazerand, P., Dignum, V., … & Vayena, E. (2018). AI4People—An ethical framework for a good AI society: Opportunities, risks, principles, and recommendations. Minds and Machines, 28(4), 689-707.
Harfouche, A. L., Nakhle, F., Harfouche, A. H., Sardella, O. G., Dart, E., & Jacobson, D. (2023). A primer on artificial intelligence in plant digital phenomics: embarking on the data to insights journey. Trends in Plant Science, 28(2), 154-184.
Jordan, M. I., & Mitchell, T. M. (2015). Machine learning: Trends, perspectives, and prospects. Science, 349(6245), 255-260.
Mehrabi, N., Morstatter, F., Saxena, N., Lerman, K., & Galstyan, A. (2021). A Survey on Bias and Fairness in Machine Learning. ACM Computing Surveys (CSUR), 54(6), 1-35.
Mittelstadt, B. D., Allo, P., Taddeo, M., Wachter, S., & Floridi, L. (2016). The ethics of algorithms: Mapping the debate. Big Data & Society, 3(2), 2053951716679679.
Montesinos-López OA, Montesinos-López A, Pérez-Rodríguez P, Barrón-López JA, Martini JWR, Fajardo-Flores SB, Gaytan-Lugo LS, Santana-Mancilla PC, Crossa J. A review of deep learning applications for genomic selection. BMC Genomics. 2021 Jan 6;22(1):19. doi: 10.1186/s12864-020-07319-x. PMID: 33407114; PMCID: PMC7789712.
Schuhl, H., Peery, J. D., Gutierrez, J., Gehan, M. A., & Fahlgren, N. (2022). Simplifying PlantCV workflows with multiple objects. Authorea Preprints.
Singh, A., Ganapathysubramanian, B., Singh, A. K., & Sarkar, S. (2018). Machine learning for high-throughput stress phenotyping in plants. Trends in Plant Science, 21(2), 110-124.
Voigt, P., & Von dem Bussche, A. (2017). The EU general data protection regulation (GDPR). A Practical Guide, 1st Ed., Springer International Publishing.
______________________________________________
About the Authors
Maneesh Lingwan is a Postdoctoral Associate at Donald Danforth Plant Science Center, and a 2024 Plantae Fellow. He is a passionate plant biochemist interested in mass spectrometry technologies, isotope mapping, and strategies to decode cell-specific metabolism. Besides research, Maneesh aims to inspire and educate younger students by providing valuable insights about STEM careers and helping them to navigate opportunities. You can find him on X: @LingwanManeesh.
Nibedita Priyadarshini is an algal biologist, and a 2024 Plantae Fellow. She has a passion for turning the world into her personal petri dish! When she’s not busy peering through microscopes or waxing poetic about the wonders of plant research, you can find her charming everyone with her quirky sense of humor and an uncanny ability to make phytohormones sound like the coolest party in town. You can find her on X: @nivi_pri